This article forms part of Eurostat’s methodology manual on territorial typologies.
Purpose of this publication
Reliable and comparable datasets for different territorial typologies can only be produced on the basis of coherent building blocks that are spatially joined, stable over time, and independent from administrative changes. Location is a key attribute to virtually all official statistics: it provides the structure for collecting, processing, storing, analysing and aggregating data. The framework provided by a specific geographic feature, such as a national border, or proximity to a coastline, is often the only feature shared by different datasets. Moreover, location is a concept that most people are comfortable with, as statistics on specific areas help people to understand the relevance of particular statistics.
Eurostat’s Methodological manual on territorial typologies (2018 edition) was principally designed as a guide for data suppliers within the European Union (EU) so that they have the necessary information to ensure coherency within their data collections. It was also intended to be of interest to users of subnational statistics in order to better understand and interpret the wide range of official statistics that are available subnationally for the EU.
The decision to make the 1st edition of the manual reflected an important milestone concerning legislative developments: an amending Regulation (EU) 2017/2391 of the European Parliament and of the Council was adopted on 12 December 2017 as regards territorial typologies (Tercet), followed on 18 January 2018 by a consolidated and amended version of Regulation (EC) No 1059/2003 of the European Parliament and of the Council on the establishment of a common classification of territorial units for statistics (NUTS). Prior to the Tercet initiative, these territorial typologies and their related methodologies didn’t have any legal basis and they were, as such, not formally recognised by the European statistical system (ESS). These issues were subsequently addressed with subnational statistics now having a legal basis that is developed around a set of impartial and transparent territorial typologies. The main objectives of Tercet included, among others
- establishing a legal recognition of territorial typologies for the purpose of European statistics by laying down core definitions and statistical criteria
- integrating territorial typologies into the NUTS Regulation so that specific types of territory could be referred to in thematic statistical regulations or policy initiatives, without the need to (re-)define terminology such as cities, urban or coastal areas
- ensuring methodological transparency and stability, by clearly promoting how to update the typologies.
The 2nd edition of the Methodological manual on territorial typologies (2024 edition) keeps the same structure, but it has been edited to present the latest legislative changes and methodological updates that have occurred since the 1st edition of the publication was released in 2018. The structure of this online manual is as follows.
- After an introduction, it provides an overview of the typologies presented, details concerning the basic building blocks (the ‘grids’) that are used to construct these typologies, and some background as to the various uses that can be made of subnational statistics.
- This is followed by an explanation of cluster types (Part A) – which are related groups of 1 km² grid cells that share the same characteristics in terms of their population density.
- The main body of the manual contains detailed explanations for the 2 main types of territorial typologies that are covered in Tercet-related legislation:
- local typologies (Part B) – which presents a range of typologies that are based on data for local administrative units (LAUs)
- regional typologies (Part C) – which presents a range of typologies that are based on regional data classified according to NUTS level 3.
- The last part presents a number of other regional typologies that aren’t currently covered by legislation (Part D), where further development work and/or agreement with other European Commission services is required before a legislative basis might be established; these typologies are also based on regional data classified according to NUTS level 3.
Overview of typologies
Most economic, social and environmental situations and developments have a specific territorial dimension – they are located in a fixed place – dependent, to some degree, upon a range of territorial resources, for example, transport or communications networks, access to services, as well as natural and human resources. Such geospatial diversity makes analysing different territories a complex task. Territorial typologies help users of official statistics to understand the diverse characteristics of the regions/territories within a country or another geographical area. By categorising territories based on common features (such as demographics, economic activities, or physical characteristics), policymakers and/or researchers can gain insights into the unique challenges and opportunities each territory faces. The typologies thus provide a systematic framework for understanding, analysing, and addressing the complex dynamics of regional development, thereby contributing to more effective and equitable spatial planning and policy implementation. In order to cast some light on territorial patterns, Eurostat has expanded its range of statistics that are published for territorial typologies.
A broad range of territorial typologies were integrated into the NUTS Regulation in December 2017, underlining the importance of subnational statistics as an instrument for targeted policymaking and a tool for understanding and quantifying the impact of policy decisions in specific territories. The Regulation provides a legal basis for the use of 1 km² grid cells, local administrative units (LAUs) and NUTS, as well as providing information as to how each of these concepts relates to establishing a complementary set of territorial typologies. By integrating these territorial typologies into a single legal text, they can be applied consistently and harmoniously, making it possible for them to be cross-referenced from other legal acts and programmes.
Key terms
There are a number of key terms which appear in several places throughout this online publication. A short definition of these key terms is provided here before going into more detail later in this introduction (see Building blocks for typologies for more detailed definitions/descriptions).
Clusters: groups of grid cells that conform to a particular criterion in relation to their population density. For example, similar grid cells may be grouped into rural grid cells, urban clusters (moderate-density clusters) or urban centres (high-density clusters).
Local administrative units (LAUs): a system for dividing up a territory for the purpose of developing statistics at a local level. These units are usually low level administrative divisions within a country, ranked below a province, region, or state.
Classification of territorial units for statistics (NUTS): a territorial classification that subdivides the territory of the EU into 3 different hierarchical levels – NUTS level 1, level 2 and level 3 respectively – detailing larger to smaller territorial units. The NUTS is based on Regulation (EC) No 1059/2003 of the European Parliament and of the Council of 26 May 2003 on the establishment of a common classification of territorial units for statistics (NUTS), which is regularly updated. A consolidated version of the latest (amended) legislative text is available on the EUR-Lex website.
Population grid: a lattice composed of 1 km² grid cells overlaying a particular territory, for which information is collected relating to the number of inhabitants. These grids are a powerful tool to describe the spatial distribution of population which may be used to analyse the interrelationships between human activities and the environment. The grid is stable over time, not dependant on changes in administrative boundaries and may be used for spatial aggregations to various territories of interest.
Region: a term with 2 distinct meanings in a statistical context. For the purpose of this publication a region refers to a subnational, geographical area based on NUTS. Although not used in this publication, a region may also refer to a supranational area, as in a region of the world (for example, Latin America, south-east Asia, or indeed the EU).
Figure 1 presents an overview of the main territorial typologies that have been developed, often in conjunction with other European Commission services and/or other international organisations. At the most basic level, these concepts can be split into 3 different groups, covering grid typologies, local typologies and regional typologies.
Grid typologies: Eurostat collects population statistics based on 1 km² grid cells. These very detailed statistics are used to establish various cluster types – namely, urban centres, urban clusters and rural grid cells based on their population figures, and – when defining the different cluster types – the contiguity of the cells.
Local typologies: based on statistics for local administrative units (LAUs) which generally comprise municipalities or communes across the EU. Statistics for LAUs may be used to establish local typologies including the degree of urbanisation (cities; towns and suburbs; rural areas); functional urban areas (cities and their surrounding commuting zones); coastal areas (coastal and non-coastal areas).
Regional typologies: statistics that are grouped according to the classification of territorial units for statistics (NUTS); they provide information at a relatively aggregated level of detail, with data presented for NUTS level 1, level 2 and level 3 regions respectively, detailing larger to smaller territorial units. Only the most detailed statistics at NUTS level 3 are used as building blocks to establish the urban-rural typology (predominantly urban regions, intermediate regions and predominantly rural regions), the metropolitan typology (metropolitan and non-metropolitan regions), the coastal typology (coastal and non-coastal regions), each of which has a legislative basis. There are 3 other regional typologies (covered in this manual) for which there is, at present, no legal basis: the border typology (border and non-border regions), the island typology (island and non-island regions) and the mountain typology (mountain and non-mountain regions). The EU also has a number of outermost regions (defined by Article 349 of the Treaty of the Functioning of the European Union (TFEU)) which are geographically distant from the European continent. As their collective legal definition is provided for by the TFEU, this typology isn’t specifically covered in this manual. That said, the outermost regions form an integral part of the EU and as such they are included – as individual regional entities – within each regional typology.
The 3 different types of territorial typologies – grid, local and regional – are closely interlinked, as they are based on the same basic building blocks, namely, classifying population grid cells to different cluster types and then aggregating this information either by LAU or by region to produce statistics for a wide variety of different typologies. Figure 2 presents an example for how urban areas in the EU are defined at 3 different – but coherent – levels
- at an initial level, urban centres (or high-density clusters) are identified as groups of grid cells with a population density of at least 1 500 inhabitants/km² and collectively a population of at least 50 000 inhabitants
- these urban centres may be superimposed onto LAUs to identify cities (LAUs where at least 50% of the population lives in an urban centre) and commuting zones (LAUs surrounding a city characterised by at least 15% of their population commuting to work in the city) – the term functional urban area is used to describe this wider aggregate that consists of a city and its surrounding commuting zone(s)
- functional urban areas may then be superimposed onto NUTS level 3 regions to identify metropolitan and non-metropolitan regions, defined as a NUTS level 3 region or groups of NUTS level 3 regions where at least 50% of the population lives inside a functional urban area that is composed of more than 250 000 inhabitants.
This brief overview concludes with a set of 10 maps that show the various territorial typologies that are presented within the main body of this publication; they underline the broad range of potential analyses that may be carried out when exploiting Eurostat’s subnational statistics.
In 2023, the population density of Poland (118 inhabitants per km²) was relatively close to the average for the whole of the EU (106 inhabitants per km²). The 1st map shows that across the Polish territory there were relatively few people living in many eastern and northern areas, while the most densely populated areas were concentrated around the capital city of Warszawa and in southern areas.
Poland displays a polycentric pattern of urban developments, its principal urban centres – composed of high-density clusters – are shown in the 2nd map, including from north to south, Gdańsk, Szczecin, Białystok, Bydgoszcz, Warszawa, Poznań, Łódź, Lublin, Wrocław, Katowice and Kraków.
The 3rd and 4th maps are based on local typologies. Map 3 highlights that relatively large swathes of Poland are rural areas with low levels of population density. The 4th map underscores that commuting zones surrounding cities in Poland are often relatively small. With the exception of the capital city, Polish cities tend to be relatively compact, with clear boundaries dividing these cities and more sparsely populated surrounding areas.
There are 2 different territorial typologies that concern maritime areas, 1 based on LAUs and the other based on NUTS level 3 regions. Map 5 shows the 1st of these detailing coastal areas in Poland, while Map 8 shows the other, detailing coastal regions.
Maps 6 to 10 present information for regional typologies based on NUTS level 3 regions. The 1st of these shows the urban-rural typology, with most Polish regions characterised as being predominantly rural or intermediate regions. There was, however, a cluster of predominantly urban regions in the south-western corner of the territory. Map 7 shows that the vast majority of metropolitan regions in Poland were located in the southern half of the county, with the most notable exception being the capital city.
The final map for regional typologies shows the 2nd typology related to maritime areas, namely, the coastal typology. This may be used to identify regions by individual sea basins, for example, regions that border the Baltic Sea, North Sea, North-East Atlantic Ocean or the Mediterranean Sea; the typology also covers outermost regions (which form an integral part of the EU).
The final pair of maps presenting the various territorial typologies shows 2 more regional typologies; note however that contrary to the typologies shown in Maps 6 to 8, the statistics collected for the typologies that are shown in Maps 9 and 10 don’t have any legal basis.
Map 9 identifies the common inland and external border regions of Poland, while there was also 1 Polish region (Miasto Szczecin) with more than 50% of its population living within 25 km of a land border.
Map 10 presents the mountain typology, which is based on regions characterised by having more than half of their population and/or surface area in mountain areas. Topographic mountain areas are defined using a range of criteria linked to height/altitude: they include all areas that are above 2 500 metres, but also include areas that are as low as 300 metres if these are characterised by a sufficiently undulating landscape (for example, the Norwegian fjords).
Note: Poland doesn’t have any island regions – however, more information on these regions, including a map with all of the EU’s island regions, is presented in Chapter 9.
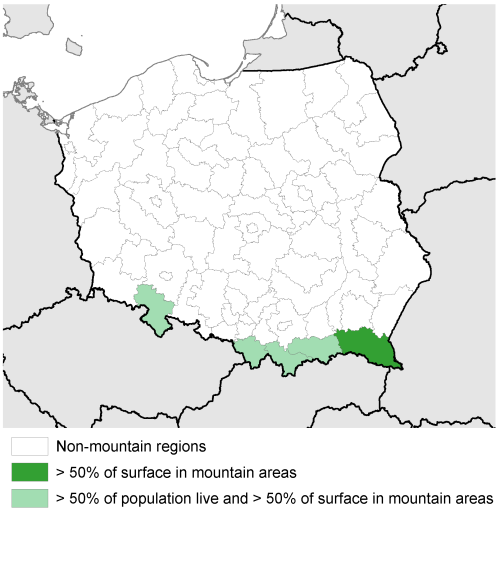
Building blocks for typologies
As noted above, the territorial typologies that have been developed by Eurostat can be split into 3 principal groups, covering grid-based typologies, local typologies based on LAUs and regional typologies based on NUTS level 3. This section provides a more detailed explanation for the 3 basic building blocks that underpin the various typologies, namely
- the population grid
- local administrative units (LAUs)
- the classification of territorial units for statistics (NUTS).
Population grid
Short description
Generally, grid data consist of statistics that are geographically referenced to a system of (usually squared) grid cells in a common reference grid with Cartesian coordinates. More specifically, when we are talking about a population grid, it is composed of grid cells containing population counts for each cell. Eurostat gives preference to the use of a 1 km² square grid that is overlaid across the EU territory. For other purposes or analyses, these grid cells could be easily aggregated to larger sizes, for example to square grids whose sides measure 5, 10, 25, or 100 km.
Why use grid statistics?
Making use of a geographical grid for displaying population densities isn’t a new idea: indeed, examples exist from the late 18th century when this approach was adopted in statistical atlases. However, it is only relatively recently that it has become possible to consider the creation of population grids over very large areas, such as the EU, in a harmonised way. With the introduction of new technologies and increased computing power, a growing number of national statistical authorities developed the ability to produce statistics for very small areas. For example, during a population and housing census, most statistical authorities are now able to capture data based on geocoded data collection points such as addresses or buildings that is usually much more detailed than any other official data they publish.
Traditionally, official statistics have been reported in accordance with a hierarchical system of administrative units ranging from local administrative units (LAUs), through regions and countries to supranational aggregates covering, for example, the whole of the EU. While these systems provide high-quality data for accounting purposes and for the respective territorial authorities at each level of the hierarchy, they aren’t so suitable for studying the causes and effects of many socioeconomic and environmental phenomena which are often independent of administrative boundaries, for example, commuting, leisure activities, flooding or weather events. When studying such phenomena, a system of grids with equal-size grid cells has many advantages
- grid cells all have the same size allowing for easy comparison
- grids are stable over time
- grids integrate easily with other (scientific) data and there is no need for corrections connected to changes in administrative units
- grid systems can be constructed/assembled to form areas that match a specific purpose or study area
- data for grid cells can be easily aggregated into any hierarchical system
- grids provide new analytical and visualisation opportunities (raster analytics in a vector environment).
The detailed nature of population grid statistics is generally considered an advantage over more traditional statistics that are based on larger administrative or statistical areas. For example, the results presented for the urban-rural typology (in Map 6 above) show a concentric pattern of decreasing population density radiating outward from the Polish capital. By contrast, the information based on the population grid (in Map 1 above) shows more vividly a distinct area of concentrated population in the capital that quite rapidly transforms into relatively low levels of population density in the surrounding areas, thereby providing a more realistic portrait of where people actually live.
Explanation of the population grid
Population grids are a powerful tool for describing the spatial distribution of a population and are particularly useful for analysing socioeconomic phenomena that are independent of administrative boundaries. This next section provides an example of how population grids work in practice.
Normally, the production of grid statistics requires the existence of geo-referenced point datasets with high spatial accuracy (in most cases these are building, business or address registers, geocoded with geographic coordinates to which the statistical information can be referenced). A population grid is composed of a set of equally sized cells that is overlaid across the territory. For European data, a 1 km² grid represents a good compromise between analytical capacity and data protection. There are 3 methodological solutions envisaged for establishing the total number of inhabitants living in each of these 1 km² grid cells.
Method 1: aggregation method
The aggregation method for producing population grid data is based on aggregating geocoded micro data (it is also called the ‘bottom-up approach’). This is the preferred method for producing population grid data, for example, aggregating a geocoded point-based data source, such as an address.
Population grid cells are referenced according to their coordinates which makes it possible to pinpoint them on a map. The example of Figure 3 shows a large number of point-based data plots – the individual blue circles – that have been overlaid onto a statistical grid; the plots represent the population at their usual place of residence.
A unique grid cell identifier (for example, CRS3035RES1000mN4626000E5034000) specifies, in accordance with INSPIRE provisions, the coordinates of each cell in relation to the reference frame. The individual parts of the code convey the following information
- ‘CRS3035’ is a unique identifier for the reference frame used in the grid (ETRS89-LAEA)
- ‘RES1000 m’ gives the resolution used in the grid (1 000 m or 1 km)
- ‘N[y]’ encodes the so-called northing value, where [y] stands for a string containing all digits of the integer y coordinate in metres of the lower-left grid cell corner
- ‘E[x]’ encodes the so-called easting value, where [x] stands for a string containing all digits of the integer x coordinate in metres of the lower-left grid cell corner.
In the example, the coordinates of each cell concern their relative position in relation to the origin (as measured in a northerly and easterly direction): for example, cell B2 concerns grid reference N4626000E5034000, while the adjacent cell C2 is referenced as N4626000E5035000. These references indicate that grid cell N4626000E5034000 identifies a 1 km² cell that has coordinates for its lower left corner of Y=4 626 km (north), X=5 034 km (east) in relation to the origin (0 km, 0 km).
This point-based information in each cell may be aggregated to produce a population count for each cell, as shown in Figure 4. For example, cell B2 has a total population count of 58 inhabitants, while in the adjacent cell (C2) the count is 52 inhabitants. The population density of each grid cell may subsequently be assigned to a population density class, as shown by the coloured shading in Figure 4: no inhabitants per km² (white background); 1 to 4 inhabitants per km² (light green shade); 5 to 19 inhabitants per km² (medium green shade); 20 to 199 inhabitants per km² (darker green shade).
The schema used for the aggregation method (or bottom-up approach) is shown in Figure 5.

Source:Jakobi, Ákos (2015) A grid: aggregált és dezaggregált rácsmodellek a területi egyenlőtlenségek vizsgálatában. TERÜLETI STATISZTIKA, 55 (4) pp. 322–38. ISSN 0018-7828. Online: 2064-8251. Available here.
Method 2: disaggregation method
In the absence of geocoded micro data, alternative approaches may be employed to produce data for the grid. The 1st of these approaches – the disaggregation method (also called the ‘top-down approach’) – uses population statistics for LAUs in combination with auxiliary spatial data. The total population count for an LAU may be disaggregated using data on land use and/or land cover to estimate the number of inhabitants that are living in each 1 km² grid cell; this may be done, for example, through the visual inspection of satellite images overlaid on the grid to determine if there are any buildings in each grid cell. Such an approach may have some limitations: for example, it is difficult to define the actual height of buildings from a satellite image and, as a consequence, the number of dwellings for each building’s footprint. This has been identified as a shortcoming, insofar as models using this approach tend to systematically underestimate the population living in densely-populated areas (where there tend to be higher buildings that may be composed of multiple dwellings) while overestimating the population of thinly populated areas (that are generally characterised by 1 or 2-storey dwellings). For more information, see
- Spatially disaggregated population estimates in the absence of national population and housing census data, N. A. Wardrop, W. C. Jochem, T. J. Bird, H. R. Chamberlain, D. Clarke, D. Kerr, L. Bengtsson, S. Juran, V. Seaman, and A. J. Tatem
- A high-resolution population grid map for Europe, F. Batista e Silva, J. Gallego and C. Lavalle
- Spatial disaggregation of population data onto Urban Footprint data, S. M. Starmans
- A volumetric approach to spatial population disaggregation using a raster build-up layer, land use/land cover databases (SIOSE) and LIDAR remote sensing data, F. J. Goerlich.
The schema used for the disaggregation method (or top-down approach) is shown in Figure 6.
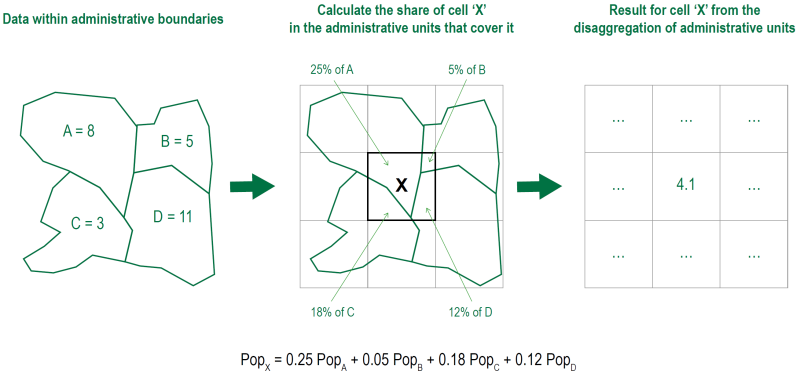
Source:Jakobi, Ákos (2015) A grid: aggregált és dezaggregált rácsmodellek a területi egyenlőtlenségek vizsgálatában. TERÜLETI STATISZTIKA, 55 (4) pp. 322–38. ISSN 0018-7828. Online: 2064-8251. Available here.
Method 3: hybrid method
The final method for producing population grid statistics is a hybrid method based on combining the aggregation and disaggregation techniques; this method provides a compromise between accuracy and the availability of data. Hybrid solutions may refer to using different source data to establish a geocoded framework, for example, combining geospatial, administrative and statistical sources.
GEOSTAT
GEOSTAT was launched by Eurostat in cooperation with the European Forum for Geography and Statistics (EFGS) in early 2010. It is a long-term programme designed to set up and promote the use of geospatial statistics including grid-based statistics through developing a methodology for official geospatial statistics in the EU, both for the EU as a whole and for individual EU countries. The initiative also aims to develop a set of common guidelines for the collection and production of population grid statistics.
In part to meet their requirements in relation to Regulation (EC) 763/2008 on the population and housing census, public administrations developed their geospatial statistics, collecting information either for LAUs or at an even more detailed level. Indeed, the census acted as a stimulus to trigger a range of initiatives for establishing geocoded building, address and population registers. As geocoded data sources with sufficient accuracy and reliability were made available, many of these were subsequently used as inputs for developing the GEOSTAT 2011 population grid as part of the GEOSTAT programme, which contains information for 29 EU and European Free Trade Association (EFTA) countries. As a result, the aggregation method was used to derive population grid statistics for 62% of the census population in 2011, while the corresponding share for the GEOSTAT 2006 population grid was 30% coverage. The geospatial framework for GEOSTAT 2011 is a standardised 1 km² grid that follows INSPIRE specifications and is based on the adoption of the ETRS89 Lambert Azimuthal Equal Area coordinate reference system. The grid is currently used in various statistical production processes, including grid-based typologies, local typologies and regional typologies. The underlying data can be downloaded and used free of charge for non-commercial purposes. While the GEOSTAT population grid covers continental Europe, as well as the Açores, Canarias and Madeira, it doesn’t extend to the remaining outermost regions of the EU. The Joint Research Centre (JRC) of the European Commission’s produced a global population grid that was used to determine regional typologies for the remaining outermost regions.
A negative consequence of developing grid-based statistics that have a much greater level of geographical detail is that there are increased concerns around data confidentiality and/or the risk of disclosure. Moreover, when introducing supplementary variables linked to the population (such as analyses by sex, by age or by type of housing) these issues may become even greater. The GEOSTAT 2011 population grid only contains information for the total number of inhabitants at their place of usual residence. This statistic was usually considered as non-sensitive by national statistical authorities which, as a result, didn’t apply any data protection methods for confidentiality issues; note however that national laws may require NSIs to protect the identification of individual citizens. For those countries that set confidentiality thresholds for GEOSTAT 2011 the minimum number of inhabitants per grid cells was 3 to 10 individuals; under this threshold the population count was suppressed.
Eurostat census population grid 2021
The 2021 population and housing census comprised a major innovation with the presentation of key census topics for an EU-wide 1 km² grid. This allows much more flexible analysis, even at a cross-border level, tailored to research or policy needs.
The data are available as a raster (GeoTIFF) file and as a geopackage (GPKG) in the European Terrestrial Reference System 1989 Lambert Azimuthal Equal Area (ETRS989-LAEA, EPSG:3035) projection. At the time of writing, a limited number of variables (for example, the population count) are available, due to ongoing data submission and validation processes. In the coming months, these datasets will be published according to INSPIRE implementing rules.
Initial results derived from the 2021 census population grid are shown in Map 11. It presents population density as measured by the number of inhabitants per 1 km2 grid cell, from uninhabited grid cells (in white) through sparsely-populated grid cells to densely-populated grid cells.
Source: Eurostat (GISCO)
Does the population grid change over time?
Population grids essentially remain stable over time unlike systems that are based on administrative boundaries (which can change each year). The grid that is currently in use by Eurostat is the census population grid of 2021.
There have been 3 previous iterations of population grids produced at a pan-European level – GEOSTAT 2006, GEOSTAT 2011 and GEOSTAT 2018. These provide an opportunity to compare results and to analyse changes over time. Note that some of the differences between the results for these grids may be linked to changes in the production methodology and in particular the fact that there was less disaggregated information used in 2011 (compared with 2006 and 2018). Furthermore, GEOSTAT 2006 covered the territory of 26 EU countries (no information for Croatia or Cyprus) and the 4 EFTA countries and was based on a total population of 502.6 million inhabitants, while GEOSTAT 2011 was extended to include information for Croatia and Cyprus, with 514.9 million inhabitants spread across 1.95 million unique grid cells, while there were 2.47 million uninhabited grid cells. The JRC’s GEOSTAT 2018 grid has an intercensal reference year with disparate population data and with variable and often coarse spatial resolution. The spatial coverage of GEOSTAT 2018 included information for 27 EU countries, the 4 EFTA countries and 7 countries in the Western Balkans (to make a total of 38).
Provisional results for the 2021 census population grid covering 27 EU countries and 3 EFTA countries (no data for Iceland) identify 2 624 362 uninhabited grid cells. By contrast, there were 1 900 292 populated grid cells (42.0% of the total count). Among these, there were 51 524 grid cells with a single inhabitant, while at the other end of the scale the highest population count for any of the 1 km² grid cells was recorded in Barcelona (Spain), at 54 163 inhabitants. Across the EU countries and 3 EFTA countries, there were 32.8 million people living in populated grid cells characterised by less than 100 inhabitants. This means that 7.2% of the population was spread over close to three quarters (72.8 ) of the populated grid. By contrast, 126 million people (or 27.5% of the population) were living in densely populated grid cells characterised by at least 5 000 inhabitants, covering just 0.8% of the populated grid.
While the most detailed building blocks – in other words, the population grid – for typologies remain stable over time (as discussed above), it is important to note that this doesn’t preclude changes to statistics that are based on it. For example, each time there are changes to the boundaries of LAUs (usually an annual exercise) or to the boundaries of NUTS regions (usually every 3 years) then changes to these classifications should be reflected in the statistics that are produced for different territorial typologies. Taking the example of the schematic overview defining urban areas in the EU (as shown in Figure 2 above), any modification to the boundaries of LAUs or regions would require the underlying information – that derived from grid-based data – to be reassessed in relation to such boundary changes.
Note: Eurostat are discussing post-2021 census developments with national statistical authorities. It is possible that from the mid-2020s onwards, the ESS will agree to produce annual counts of populations (based on the usual place of residence) for a 1 km² grid, with data to be made available within 12 months of the end of the reference period.
Which territorial typologies are impacted by changes to the population grid?
Those territorial typologies impacted by changes to the population grid can be identified by referring to Figure 1 above. It shows that population grid statistics for 1 km² cells are used directly to classify groups of grid cells into the following cluster types: urban clusters, urban centres and rural grid cells (see Chapter 1 for more details on cluster types).
This link to cluster types is particularly important insofar as these statistics are themselves used as building blocks for developing basic territorial typologies such as the degree of urbanisation (see Chapter 2) or the urban-rural typology (see Chapter 5). Information for urban clusters and urban centres is also used as a building block for developing urban typologies, with these statistics forming the basis – as already shown in Figure 2 – for data on cities and their commuter zones (see Chapter 3) as well as statistics on metropolitan regions (see Chapter 6).
Further information
Glossary entries: population grid cell
Detailed methodology: GEOSTAT 2011
Dedicated section: Gisco
Other information sources: European Forum for Geography and Statistics (EFGS) and INSPIRE – infrastructure for spatial information in Europe
Published information
Visualisation tools: Eurostat publishes datasets for population grids through its website, where there are also a range of tools for visualising the information.
Download data: the GEOSTAT datasets can be accessed and used for non-commercial purposes. The data are available on Eurostat’s website, here. GEOSTAT data are provided as shapefiles, geospatial vector data which is quasi-standard in the world of geographic information systems (GIS); indeed, almost any commercial or open source GIS software should be able to process shapefiles. Note that there are quite specific rules concerning the licensing conditions for these datasets that govern access, conditions and restrictions of use.
Local administrative units
Short description
Local administrative units (LAUs) are used to divide up the territory of the EU for the purpose of providing statistics at a local level. They are low level administrative divisions of a country below that of a province, region or state. Not all countries classify their locally governed areas in the same way and LAUs may refer to a range of different administrative units, including municipalities, communes, parishes or wards.
What are local administrative units?
Administrative divisions are generally the oldest nomenclatures for territorial units provided for by law, as they delineate local authorities with representative bodies. These administrative units are – depending upon the degree or centralisation/autonomy of political systems – charged with fulfilling the needs of local communities, for example, socioeconomic development, spatial planning, utilities, culture or the environment.
Such administrative divisions usually exist at different hierarchical levels (although some levels may not exist in smaller EU countries), ranging from regional and/or county/state administrations, through districts and/or local councils, down to municipalities/communes. It is this collection of units at the bottom of the administrative hierarchy that is used to define LAUs. LAUs implement policies and are considered as appropriate building blocks for constructing local level typologies, such as statistics for the degree of urbanisation, functional urban areas and coastal areas.
Up until 2016, there were 2 different levels of LAU
- LAU level 1 (formerly NUTS level 4) which was defined for most, but not all of the EU countries
- LAU level 2 (formerly NUTS level 5) which consisted of municipalities/communes or equivalent units across all EU countries.
Since 2017, a single level of LAU has been kept. It is important to note that existing administrative units within the EU countries constitute the 1st criterion used to define LAUs. This means there are considerable differences across the countries between the naming conventions and concepts used. Indeed, Regulation (EC) No 1059/2003 on the establishment of a common classification of territorial units for statistics (NUTS) defines an administrative unit as: a geographical area with an administrative authority that has the power to take administrative or policy decisions for that area within the legal and institutional framework of the country. The distinct administrative divisions used for each country are defined within Annex III.
Country | Administrative division |
---|---|
Belgium | Gemeenten/Communes |
Bulgaria | Общини (Obshtini) |
Czechia | Obce |
Denmark | Kommuner |
Germany | Gemeinden |
Estonia | Linn, vald |
Greece | Δήμοι (Dimoi) |
Spain | Municipios |
France | Communes |
Croatia | Gradovi, općine |
Ireland | Local electoral areas |
Italy | Comuni |
Cyprus | Δήμοι, κοινότητες (Dimoi, koinotites) |
Latvia | Valstspilsētas, novadi |
Lithuania | Savivaldybės |
Luxembourg | Communes |
Hungary | Települések |
Malta | Localities |
Netherlands | Gemeenten |
Austria | Gemeinden |
Poland | Gminy |
Portugal | Freguesias |
Romania | Municipii, Orașe and Comune |
Slovenia | Občine |
Slovakia | Obce |
Finland | Kunnat/Kommuner |
Sweden | Kommuner |
To give an idea of the variations that may exist between these national concepts for LAUs, in 2023 there were 34 956 LAUs identified in France, compared with 10 993 in Germany, 7 904 in Italy and less than 100 in Denmark, Malta and each of the Baltic countries; these differences reflect, to a large degree, the organisation of local government/representation in each EU country.
Furthermore, there are often sizeable differences between LAUs within the same EU country in terms of their number of inhabitants or the area that they cover. In 2023, there was a single person living in the French commune of Rochefourchat in the south-west of France, while there were as many as 2.2 million inhabitants living within the commune of Paris. In Spain, the boundaries of the smallest municipality – Llocnou de la Corona in the Comunitat Valenciana – delineated an area of just 0.0126 km². This was in stark contrast to the area covered by the largest municipality – the city of Cáceres in the western region of Extremadura – some 1 750 km².
Encoding administrative divisions within the national territory is an essential task of national statistical systems, assigning an alphanumerical code to the various levels. This makes it easier for national statistical authorities to provide a wide range of subnational statistics, often at a highly disaggregated level of detail, in an attempt to meet the growing need for socioeconomic information at a local level. As well as being a basis for statistical analysis in their own right, LAUs are also used as 1 of the principal building blocks to produce data for regions and for other territorial typologies.
Why does the list of local administrative units change?
To meet the increased demand for statistics at a local level, Eurostat maintains a list of LAUs. This tracks any changes that take place: some EU countries make frequent changes to their LAUs while others almost never change them. Article 4 of the NUTS Regulation (EC) No 1059/2003 provides details of how this system should be managed.
- During the 1st half of each calendar year, the countries should provide details of any changes to local administrative units with reference to 31 December of the previous year.
- Eurostat is responsible for amending, on an annual basis, the complete list of LAUs on the basis of changes to administrative units that have been communicated to it by the countries.
- Eurostat should publish the revised list of LAUs by the end of each calendar year.
Guidelines for developing LAU lists
- A LAU code is the key used for correspondence with all related territorial typologies.
- There are no coding conventions across the EU for LAUs, national codes are employed.
- In the case of LAU closures, old codes mustn’t be re-used or maintained.
- LAU codes should be provided as alphanumeric strings rather than numbers.
To amend the LAU list on an annual basis, Eurostat introduced a new transmission format in 2018. As well as detailing changes to LAUs it also seeks to integrate information on changes to territorial typologies for LAU-based classifications – the degree of urbanisation, functional urban areas and coastal areas – which are directly impacted by any LAU boundary changes. As such, the LAU list is managed together with local typologies in order to align correspondence tables at the same time. This single procedure makes it possible for Eurostat to publish annual updates for territorial typologies together with the annual LAU list at the end of each year.
Which typologies are impacted by changes to LAUs?
At a local level (for LAUs), the following typologies are based on LAUs
- degree of urbanisation – cities, towns and suburbs, rural areas (see Chapter 2)
- functional urban areas – cities and their surrounding commuting zones (see Chapter 3)
- coastal and non-coastal areas (see Chapter 4).
As such, any changes made to LAU boundaries need to be checked to see if they impact on local level typologies and, where necessary, the correspondence tables for these typologies should be updated. Concerning the datasets compiled using these, any modifications to the typologies caused by changes to the list of LAUs can be implemented in 1 of 2 different ways: applying the specific methodology for each data collection to the new LAU boundaries; or applying a simpler approach that doesn’t use geographical information systems to estimate the resulting statistics based on changes to LAU boundaries. The 1st approach is more labour intensive, while the 2nd is particularly suitable if boundary changes for LAUs are relatively small or consist principally of merging LAUs. A practical example of how this may be done is presented for the degree of urbanisation in Chapter 2 (under the heading, Changes over time that impact on the classification).
As well as providing basic statistics in their own right (for population and area) and serving as the building block for local level typologies, LAUs are also used as a building blocks for regions, as described in Article 4 of the NUTS Regulation: In each EU country, local administrative units (LAU) shall subdivide NUTS level 3 into 1 or 2 further levels of territorial units. Map 12 provides an example for part of Czechia showing how its LAUs (identified by the grey borders) are aggregated to NUTS level 3 regions (identified by the light blue borders); note the interesting case of the capital city of Praha that is both an LAU and a NUTS level 3 (and indeed a NUTS level 2) region.
Further information
Statistics Explained glossary entry: Local administrative unit (LAU)
Dedicated section: Local administrative units (LAUs)
Download data: the NUTS Regulation requires EU countries to send lists of their LAUs to Eurostat each year. This information may be supplemented by additional administrative data for the population and the total area of each LAU. These lists are published each year on the Eurostat website.
NUTS
NUTS, the classification of territorial units for statistics, is a geographical classification subdividing the territory of the EU into regions at 3 different levels – NUTS level 1, level 2 and level 3 (moving from larger to smaller territorial units). The legal basis for NUTS is provided for Regulation (EC) No 1059/2003, hereafter referred to as the NUTS Regulation. A consolidated version (including subsequent amendments) is available here.
What is the NUTS classification?
The NUTS classification is a hierarchical system for dividing up the territory of the EU for the purpose of
- the collection, development and harmonisation of EU regional statistics
- socioeconomic analyses of the regions
- NUTS level 1: major socioeconomic regions
- NUTS level 2: basic regions for the application of regional policies
- NUTS level 3: small regions for specific diagnoses
- framing EU regional policies
- regions eligible for support from cohesion policy have been defined at NUTS level 2
- the majority of the analyses made within European Commission cohesion reports are presented for NUTS level 2 regions.
The NUTS classification is set out in Annex I of Regulation (EC) No 1059/2003. It is a hierarchical classification that ascribes a specific code and name to each territorial unit and subdivides the EU countries into NUTS level 1 territorial units, each of which is subdivided into NUTS level 2 territorial units, these in turn each being subdivided into NUTS level 3 territorial units. Note that a particular territorial unit may be classified at several NUTS levels – for example, the German capital city of Berlin is coded as DE3 (NUTS 1), DE30 (NUTS 2) and DE300 (NUTS 3), all of which cover the same area.
The diagram below shows the hierarchical structure of NUTS, moving from the national territory of Germany (DE) through progressively more detailed levels of NUTS. At NUTS level 1, the German regions are aligned with the Länder, for example, Baden-Württemberg (DE1) and Bayern (DE2). Each NUTS level 1 region is subsequently subdivided into NUTS level 2 regions, for example, Bayern is split into Oberbayern (DE21), Niederbayern (DE22), Oberpfalz (DE23), Oberfranken (DE24), Mittelfranken (DE25), Unterfranken (DE26) and Schwaben (DE27). In a similar vein, NUTS level 2 regions may be subdivided into the most disaggregated regional units, as defined by NUTS level 3, for example, some of the 14 different level 3 regions within Schwaben include Ostallgäu (DE27B), Unterallgäu (DE27C) and Oberallgäu (DE27C).
The current version of the NUTS classification is NUTS 2024. This amendment came into force on 13 April 2023 and applies to the transmission of data (to Eurostat) from 1 January 2024 onwards. It covers 92 regions at NUTS level 1, 244 regions at NUTS level 2 and 1 165 regions at NUTS level 3.
Note: the NUTS classification is defined only for the EU countries. Eurostat, in agreement with the countries concerned, also has a classification with codes and labels for statistical regions of countries that aren’t members of the EU, but which are
- candidate countries awaiting accession to the EU, or
- potential candidates, or
- EFTA countries.
At the time of writing (spring 2024), agreements were in place for the classification of regional statistics with Iceland, Liechtenstein, Norway, Switzerland, Montenegro, North Macedonia, Albania, Serbia, Türkiye, Ukraine and Kosovo [1].
History of the NUTS classification
At the beginning of the 1970’s, Eurostat set-up the NUTS classification as a single, coherent system for dividing up the EU’s territory in order to produce regional statistics. For around 30 years, the implementation and updating of the NUTS classification was managed under a series of ‘gentlemen’s agreements’ between the EU countries and Eurostat. Work on Regulation (EC) No 1059/2003, to give NUTS a legal status, started in spring 2000; it was adopted in May 2003 and entered into force in July 2003.
The NUTS Regulation specifies that there should be stability in the classification to ensure that data refers to the same regional unit (considered crucial for time series statistics). However, sometimes national interests require changes to the classification of a territory and when this happens, the EU country concerned informs the European Commission about the changes. The European Commission, in turn, amends the classification at the end of each predefined period of stability.
Principles and characteristics used in the NUTS classification
The development of the NUTS classification is based on 3 underlying principles.
Principle 1: population thresholds
The NUTS Regulation defines minimum and maximum population thresholds for the size of NUTS regions (see Table 1); for the purpose of the Regulation, the population of each territorial unit consists of those people who have their usual place of residence in the area concerned.
If the total population of an EU country is below the minimum threshold for a given NUTS level, then the whole of that country shall be covered by a single territorial unit for the level in question. For example, Cyprus and Luxembourg are both covered by single territorial units at each NUTS level (1, 2 and 3).
For NUTS regions that are based on administrative levels, it is sufficient if the average size of the corresponding regions lies within the thresholds; in case of regions not based on administrative levels, each individual region should do so. However, exceptions do exist in case of geographical, socioeconomic, historical, cultural or environmental circumstances. For example, as of 1 January 2023 the population of 32 NUTS level 1 regions was below the minimum threshold of 3 million inhabitants. This group of 32 regions included 7 EU countries (Estonia, Cyprus, Latvia, Lithuania, Luxembourg, Malta and Slovenia). It also included 8 German Länder, 4 regions in France (including Régions Ultrapériphériques Françaises and the island of Corse), 3 out of the 4 regions in Greece (the exception being the capital region of Attiki), 2 out of the 3 regions in Hungary (the exception being the capital region of Közép-Magyarország), the Portuguese autonomous regions of Madeira and Açores, the Spanish and Finnish island regions of Canarias and Åland, as well as a single region from each of Belgium, the Netherlands, Austria and Sweden. As a result, despite the aim of ensuring that regions of comparable size all appear at the same NUTS level, each level may contain regions which differ greatly (at least in terms of their total population size).
Principle 2: NUTS favours administrative divisions
As noted above, for practical reasons the NUTS classification generally mirrors the territorial administrative divisions of each EU country. In doing so, this supports the availability of data and the capacity to implement policy developments. If no administrative units exist for a given level, they should be constituted by aggregating smaller administrative units to the higher level. The aggregation must respect existing administrative divisions (at the higher level) and shall take geographical, socioeconomic, historical, cultural, or environmental circumstances into consideration. The resulting aggregated units are referred to as ‘non-administrative units’.
Principle 3: regular and extraordinary amendments
The NUTS classification can be amended: the Regulation specifies under regular circumstances the classification should remain (unchanged) for a period of at least 3 years. Note however that additional amendments to the NUTS classification may take place for exceptional circumstances, for example, when countries join/leave the EU, or if there is a substantial reorganisation of the administrative structure of an EU country (as happened in Portugal in 2014). In the case of either regular or extraordinary amendments to the NUTS classification, the country concerned should replace its historical data by time series according to their new regional classification within a period of 2 years.
Which typologies are impacted by changes to NUTS?
As such, any changes made to NUTS level 3 boundaries need to be checked to see if they impact on the regional typologies (applying again any rules for determining classifications to the new NUTS boundaries), with updates to the NUTS classification reflected in correspondence tables for each of the regional typologies.
Regionally (using NUTS level 3 regions), the following typologies have a legal basis
- the urban-rural typology – predominantly urban regions, intermediate regions, rural regions (see Chapter 5)
- the metropolitan typology – metropolitan regions and non- metropolitan regions (see Chapter 6)
- the coastal typology – coastal and non-coastal regions (see Chapter 7).
Note there are 3 additional regional typologies (also based on NUTS level 3 regions) for which there is (currently) no legal basis
- the border typology – border and non-border regions (see Chapter 8)
- the island typology – island and non-island regions (see Chapter 9)
- the mountain typology – mountain and non-mountain regions (see Chapter 10).
Further information
Statistics Explained glossary entry: NUTS
Legislation: on the Eurostat website
Dedicated section: NUTS
Excel file: Correspondence table for NUTS 2024 level 3 regions and territorial typologies
Published information
Visualisation tools: Eurostat publishes data in the form of maps that are based on NUTS regions through Regions and cities illustrated (RCI). The different levels of the NUTS classification may be viewed through the Statistical atlas; the example below shows NUTS level 3 regions principally in Germany.
Maps: in *.PDF format presenting the different NUTS levels are available on Eurostat’s website.
Database: Eurostat’s website provides regional statistics by NUTS for 16 separate domains covering a wide range of socioeconomic data. These statistics are available for the following areas – demography, education, health, the labour market, labour costs, poverty and social exclusion, crime, economic accounts, structural business statistics, business demography, tourism, the digital economy and society, science and technology, transport, agriculture, the environment and energy. The data may be found: here.
Using data on territorial typologies
Eurostat publishes regional EU statistics across a broad range of statistical domains: these statistics are widely used in the context of EU regional policy. Through the Tercet initiative, the European Commission has defined territorial typologies in cooperation with the OECD, establishing legal recognition for these typologies by integrating them into the NUTS Regulation and its implementing provisions, thereby promoting a set of harmonised definitions that are based on methodological transparency, core definitions, and established criteria for creating and updating each typology (as required). The Tercet initiative therefore aims to improve the comparability and stability of these territorial typologies and has been designed to impact on the compilation and dissemination of EU subnational statistics. In turn, this has made it possible for those developing thematic statistical and policy-based regulations to refer directly to the territorial typologies when they instigate new areas for collecting or analysing subnational statistics.
The integration of a broad range of territorial typologies into the NUTS Regulation in December 2017, underlines the importance of subnational statistics as an instrument for targeted policymaking and a tool for understanding and quantifying the impact of policy decisions for specific types of territories. As shown in Maps 1 to 8 these cover several different territorial typologies for which data are now available across the EU at different levels – grid typologies, local typologies and regional typologies. The availability of these typologies and related data has, in turn, stimulated policymakers to ask questions such as: does it make sense to have the same policy target for pollution in a city centre as in an area of natural beauty? Or, does it make sense to have the same policy target for educational attainment in a capital city as in a remote, sparsely-populated rural area?
Analyses such as these have led to a territorial dimension being introduced into a range of EU policy areas and their related statistics. Grouping different types of regions and/or areas according to territorial types can help in understanding common patterns, for example, urban areas/regions generally perform better in economic terms and may act as hubs for innovation and education; at the same time, they may also be characterised by a range of different challenges such as congestion, pollution or housing problems.
Indeed, while some of the most pressing challenges facing the EU – for example, globalisation, climate change or poverty and social exclusion – have traditionally been approached through broad sectoral policies, often implemented across the EU, policymakers have more recently analysed spatial developments for these challenges at a much more disaggregated level of detail between different types of territory both within and across EU countries; more details are provided below.
Cohesion policy
Across the EU, cohesion policy is embedded within overall economic policy coordination, in particular the European Semester, the digital transition, A European Green Deal and promotion of the European Pillar of Social Rights. These links between cohesion policy and broader policy actions have been strengthened such that regional funding to any EU country which doesn’t comply with the EU’s economic rules may be suspended.
The EU’s cohesion policy is designed to promote harmonious development by strengthening economic, social and territorial cohesion. These goals are identified in the EU treaty and are an important expression of solidarity with the poorer/weakest regions. By doing so, cohesion policy aims to promote job creation, business competitiveness, economic growth, social inclusion and sustainable development, to impact the overall quality of life across the EU.
The bulk of cohesion policy funding is concentrated on less developed EU regions with the goal of helping them to catch up and to reduce the economic, social and territorial disparities that exist across the EU. Although cohesion policy covers every region of the EU, most of the funds are targeted at regions where gross domestic product (GDP) per inhabitant is less than 75% of the EU average. By investing in key sectors like education or innovation, cohesion policy funds projects that, for example, improve transportation networks or boost small and medium-sized enterprises, thereby fostering regional competitiveness and ensuring more balanced economic development.
Cohesion policy helps to deliver many of the EU’s policy objectives. It complements the European Commission’s Political guidelines 2024–2029, while helping to deliver more specific policies such as those dealing with education, employment, energy, the environment, the single market or research and innovation.
EU cohesion policy is based on 7-year funding periods. During the period 2021–27, the framework for regional development and cohesion policy focuses on 5 key objectives
- a smarter Europe achieved through innovation, digitalisation, economic transformation and support to SMEs
- a greener, carbon-free Europe, implementing the Paris Agreement and investing in the energy transition, renewables and the fight against climate change
- a better connected Europe, with strategic transport and digital networks
- a more social Europe, supporting social inclusion, equal access to healthcare, quality employment, education and skills, while delivering on the European Pillar of Social Rights
- a Europe closer to citizens based on locally led strategies and sustainable urban development.
A total of €392 billion has been included in the multiannual financial framework for regional development and cohesion between 2021 and 2027. For more information, including a breakdown of allocations by fund and by EU country, see 2021–27: initial cohesion policy EU budget allocations. These EU allocations are supplemented by national allocations and are thereafter translated into detailed investment plans through the formalisation of national partnership agreements and detailed national and regional programmes.
Cohesion policy is delivered through a number of specific funds. They are the main financial instruments used to promote economic, social, and territorial cohesion across the EU
- the European Regional Development Fund
- the Cohesion Fund
- the European Social Fund Plus
- the Just Transition Fund (JTF).
The European Commission doesn’t allocate funding expressly to NUTS level 2 regions, but rather to ‘categories of region’ and to ‘programmes’. Statistics from regional accounts are used in the allocation of cohesion policy funds, with the NUTS classification providing the basis for regional boundaries and geographic eligibility. During the period 2021–27, eligibility for cohesion policy funds is based on NUTS level 2 regions being ranked and split into 3 groups
- less developed regions, where GDP per inhabitant was less than 75% of the EU average
- transition regions, where GDP per inhabitant was 75–100% of the EU average
- more developed regions, where GDP per inhabitant was more than 100% of the EU average.
The funding is distributed based on the economic status of different regions, with a strong emphasis on supporting less-developed areas that are most in need of investment to catch up with more prosperous regions. Although cohesion policy covers every NUTS level 2 region in the EU, most of the funds are targeted at less developed and transition regions. These regions – predominantly located in the south or the east of the EU, the Baltic countries and several outermost regions – benefit from 90% of the ERDF and ESF+ resources.
For the 2021–27 period, the allocation of cohesion policy funds continues to be largely based on regional GDP per inhabitant in purchasing power standards (PPS). While GDP per inhabitant is the primary factor used to determine the amount of funding each region receives, a new set of criteria have been added for the 2021–27 funding period to reflect better the social, economic and environmental challenges faced by different regions of the EU. These include
- youth unemployment
- low education levels
- climate change
- demographic developments
- the reception and integration of migrants.
Cohesion policy funds are delivered through shared management and partnership, a process which involves EU, national, regional and local authorities, as well as social partners and organisations from civil society (representative and community groups that are independent of government or business). The process is designed to ensure that the funds are used effectively and transparently to achieve the policy's objectives of reducing regional disparities and promoting sustainable development.
The following steps take place in the delivery process
- allocating funding and planning
- management and implementation
- monitoring and reporting
- evaluation and adjustment.
Sustainable development goals
Sustainable development may be defined as economic growth and social progress that meets the needs of present generations without jeopardising future generations. It provides a comprehensive approach bringing together economic, social and environmental considerations in ways that mutually reinforce each other.
The United Nations (UN’s) 2030 Agenda for Sustainable Development, adopted by world leaders in 2015, represents a global sustainable development framework based around 17 Sustainable Development Goals (SDGs) and 169 specific targets. It is a commitment to eradicate poverty and achieve sustainable development by 2030 worldwide, ensuring that nobody is left behind.
European policymakers recognise that coherent and integrated regional policy should form an essential part of the EU’s implementation strategy for the 2030 Agenda, whereby SDG indicators have to capture problems at a scale where they occur (the regional, sub-regional and city-level). The EU is fully committed to be at the forefront of implementing the UN’s 2030 Agenda. In November 2016, the European Commission outlined its strategic approach in a Communication, Next steps for a sustainable European future: European action for sustainability (COM(2016) 739 final).
The European Green Deal adopted in December 2019 provided new impetus for climate policy. The European Climate Law (Regulation (EU) No 2021/1119) adopted in June 2021 sets legally-binding targets for the EU to reach climate neutrality by 2050 and to reduce net greenhouse gas emissions by at least 55% by 2030 (compared with 1990 levels).
Since 2020, every European Commission work programme has put the SDGs at the heart of EU policymaking, with a Joint Declaration on legislative priorities for 2023 and 2024 including a commitment ‘to accelerate the implementation of the United Nations 2030 Agenda for sustainable development’.
In 2023, the EU conducted a voluntary review of the implementation of the 2030 Agenda for sustainable development. It identified that more progress was required, in particular for SDGs related to the protection and sustainable use of natural resources. Eurostat releases an annual monitoring report on progress towards the SDGs in an EU context.
The EU’s Urban Agenda
In May 2016, at a meeting of EU ministers responsible for urban matters held in Amsterdam, an agreement was reached on an Urban Agenda for the EU, as established by the Pact of Amsterdam. Its objective is to include an urban dimension in EU policies, and the Agenda’s implementation should lead to better regulation, better funding and better knowledge for cities in Europe. The work in ‘partnerships’ was initially focused on 14 priority areas covering the inclusion of migrants and refugees; air quality; urban poverty; housing; the circular economy; jobs and skills in the local economy; climate adaptation; energy transition; sustainable land use; urban mobility; digital transition; public procurement, security in public space; culture and cultural heritage.
In 2021, a renewal process for the Agenda was started, in line with directions provided by the New Leipzig Charter – the transformative power of cities for the common good in 2020 and its implementing document, leading in November 2021 to the Ljubljana agreement. During this 2nd phase of the Urban Agenda for the EU, 4 new partnerships have been launched so far: on sustainable tourism and greening cities (in October 2022) and on food and cities of equality (in November 2023).
Through a series of dedicated partnerships which involve – on a voluntary and equal basis – cities, EU countries, the European Commission and stakeholders such as businesses or non-governmental organisations (NGOs), work programmes and actions are designed to successfully tackle the principal challenges that are facing cities as well as contributing towards smart, sustainable and inclusive growth. For more information, see the website of the European Commission.
Urban development in the EU
The various dimensions of urban life – economic, social, cultural and environmental – are closely inter-related. Successful urban developments are often based on coordinated/integrated approaches that seek to balance these dimensions through a range of policy measures such as increasing education opportunities, urban renewal, preventing crime, encouraging social inclusion or encouraging environmental protection.
Among the EU’s 5 principal policy objectives, the urban dimension is explicitly mentioned 3 times.
- For the objective of ‘a greener, low-carbon Europe’ it is mentioned in relation to
- ‘enhancing protection and preservation of nature, biodiversity, and green infrastructure, including in urban areas, and reducing all forms of pollution’ as well as
- ‘promoting sustainable multimodal urban mobility, as part of transition to a net zero carbon economy’.
- For the objective of ‘a Europe closer to citizens’ it is mentioned in relation to ‘fostering the integrated and inclusive social, economic and environmental development, culture, natural heritage, sustainable tourism, and security in urban areas’.
During the period 2021–27, the ERDF’s investment priorities include
- promoting low-carbon strategies for all types of territories, in particular for urban areas, including the promotion of sustainable multimodal urban mobility and mitigation-relevant adaptation measures
- taking action to improve the urban environment, to revitalise cities, regenerate and decontaminate brownfield sites (including conversion areas), reduce air pollution and promote noise-reduction measures
- providing support for physical, economic and social regeneration of deprived communities in urban and rural areas.
The urban dimension of cohesion policy has been strengthened for the period 2021–27
- nationally, a minimum of 8% of the ERDF is dedicated to sustainable urban development strategies
- a new European Urban Initiative (EUI) was launched in the 3rd quarter of 2022 with the goal of supporting cities to innovate, access knowledge and reinforce the capacity for policy development
- the European Commission estimates that €24.4 billion from the ERDF will be allocated to sustainable urban development during the period 2021–27.
Rural development in the EU
There are also considerable differences between EU countries as regards their urban-rural territorial divisions. Some countries – for example, Ireland, Sweden or Finland – are very rural in character. By contrast, the Benelux countries and Malta have a high degree of urbanisation. Equally, within individual countries there can be a wide range of different typologies, for example, the densely populated, urbanised areas of Nordrhein-Westfalen in western Germany may be contrasted with the sparsely populated, largely rural areas of Brandenburg or Mecklenburg-Vorpommern in north-eastern Germany.
EU rural development policy is designed to help rural areas and regions meet a wide range of economic, social and environmental challenges; it complements the system of direct payments to farmers and measures to manage agricultural markets. The European agricultural fund for rural development (EAFRD) provides finance for the EU’s rural development policy, which is used to improve the competitiveness of agriculture, encourage the sustainable management of natural resources and climate action, achieve a balanced territorial development of rural economies and communities.
For the period 2021–27, the EAFRD has been allocated € 95.5 billion. The EAFRD is intended to help develop farming and rural areas, by providing a competitive and innovative stimulus, at the same time as seeking to protect biodiversity and the natural environment. As with other structural and investment funds, rural development policy is based on the development of multiannual partnership and operational programmes which are designed nationally/regionally by individual EU countries.
A long-term Vision for EU’s Rural Areas – Towards stronger, connected, resilient and prosperous rural areas by 2040 and its associated rural action plan are designed to help rural areas meet a wide range of economic, social and environmental challenges. Under this long-term vision, the European Commission has also proposed a Rural Pact, which is a framework for cooperation that facilitates interaction on rural matters between public authorities.
Notes
- ↑ The designation of Kosovo is without prejudice to positions on status, and is in line with UNSCR 1244/1999 and the ICJ Opinion on the Kosovo Declaration of Independence.
Explore further
Thematic section
Methodology
External links
Legislation
- Regulation (EC) No 1059/2003 – Regulation of the European Parliament and of the Council of 26 May 2003 on the establishment of a common classification of territorial units for statistics (NUTS)
- Regulation (EU) 2017/2391 – amending Regulation (EC) No 1059/2003 as regards the territorial typologies (Tercet)
- Regulation (EC) 763/2008 – Regulation of the European Parliament and of the Council on population and housing censuses
- Regulation (EU) No 1303/2013 – Regulation of the European Parliament and of the Council laying down common provisions on the European Regional Development Fund, the European Social Fund, the Cohesion Fund, the European Agricultural Fund for Rural Development and the European Maritime and Fisheries Fund and laying down general provisions on the European Regional Development Fund, the European Social Fund, the Cohesion Fund and the European Maritime and Fisheries Fund and repealing Council Regulation (EC) No 1083/2006
- Regulation (EU) No 522/2014 – Commission Delegated Regulation supplementing Regulation (EU) No 1301/2013 of the European Parliament and of the Council with regard to the detailed rules concerning the principles for the selection and management of innovative actions in the area of sustainable urban development to be supported by the European Regional Development Fund