The future of human-machine interaction at the SI-CODE FET Open project
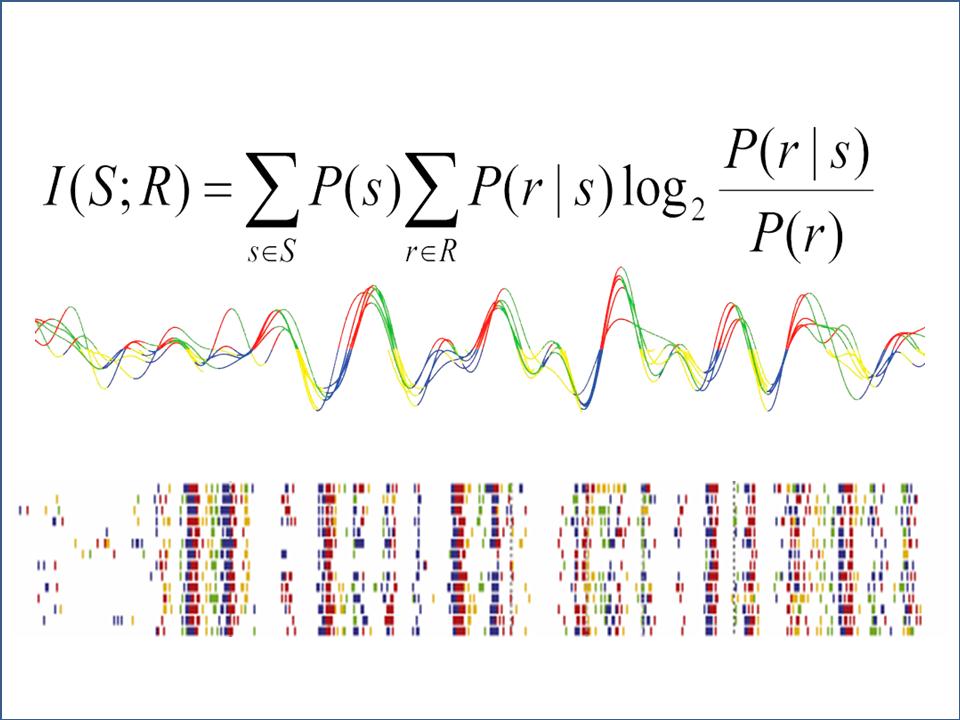
Related topics
ICT Research & Innovation Innovation Biotechnology Future and emerging technologies Italy FET Opendate: 13/11/2014
Project: Towards new Brain-Machine Interfaces: st...
acronym: SI-CODE
Project coordinator: Stefano Panzeri
See also: CORDIS
Professor Stefano Panzeri is a mathematical neuroscientist at the Center for Neuroscience and Cognitive Systems in the "Istituto Italiano di Tecnologia." His research focusses on how circuits of neurons in the brain process information and he coordinates the SI-CODE project. In this article, Prof. Panzeri explains in detail this thrilling research.
BMIs are devices mediating communication between the brain and the external world, representing both biomedical solutions and brain research tools. In the first case, BMIs may be used to restore motor or sensory functions to people who lost them due to illness or injury, while in brain research BMIs give neuroscientists a way for gaining a deeper understanding of neural information processing through the controlled interaction between neural populations and a virtually unlimited variety of external devices.
Yet, there is a most severe obstacle to improve BMI performance and bring it to the level needed for a major impact on both healthcare and basic science: neural activity appears very noisy. If human or animal subjects experience a certain sensory stimulus or execute a given motor task in an experiment, the neural activity mediating or expressing these functions will never be the same across different repetitions of the same experiment, but it will vary profoundly from one repetition the next. Indeed the most serious bottleneck in performance of the current BMIs stems from the limited amount of information that can be extracted from neural activity. This problem limits at the source the reliability and the number of the different operations that can be accomplished by such devices.
The potential solution that we conceived stems from my own experience with extracting information from the activity of groups of neurons. When I moved to neuroscience coming from statistical quantum physics 15 years ago, I was struck and fascinated by how noisy neural responses were, and how difficult it was for scientists to extract large amounts of information from the electrical activity of neurons. The prevalent view of the neuroscience community at the time was (and still in part is) that the brain manages to perform interesting computations in spite of its unreliable computing elements by averaging away the noise of each individual neuron by pooling together the messages of many different neurons. This view has always struck me as odd: the neurons are after all, the basic computing elements of the most sophisticate organ of the universe. What would be the computational advantage to build such a complex organ on unreliable foundations? The fact that this proposal cannot work becomes immediately apparent to scientists – like myself - that investigate how to decode the activity of many neurons: the information in the activity of many simultaneously observed neurons is often just marginally larger than the information carried by the best neuron in the population. This is because the noise, or variability in neural responses, is shared across the neurons, probably because it originates from a limited number of shared sources. Given that all or most neurons share the same noise, this noise cannot be discounted by pooling different neurons. Neural noise can only be discounted after individuating the origin of variability and subtracting it out.
The hypothesis that we investigate is that a large part of what appears at first sight to be neural noise reflects instead the fact that the activity of neurons does not depend only on the external variables that scientists control and manipulate (such as the presentation of a sensory stimulus or the performance of a certain task) but also on the internal “state” of the individual (for example, her/his level of attention, of motivation or of arousal) which is in turn reflected in the internal state of the network of neurons under consideration. For example, the activity of neurons in the cerebral cortex undergoes continually intrinsically generated changes in the state of the excitability of the network – visible for example as spontaneous “ups” and “downs” of neuronal activity - even when the subject is at rest.
Our key idea on how to improve communication between brains and machines stems from this example: given that the neural activity in relation to the external events depends on the state of the network, the messages carried by neurons can only be made noiseless and understood if we estimate in real time the state of neural network. The real-time state of the network, therefore, is a variable that we have to discount and eliminate from the signal we usually register. It is tempting to hypothesize that neurons in the brain must work in this way too, or in other words use state-dependent decoding algorithms to communicate.
To address this issue, the multidisciplinary SI-CODE European project has brought together empirical neuroscientists recording neural activity in vivo; mathematical neuroscientists like myself developing algorithm to model and decode neural activity; and engineers expert in the development of artificial devices for communication with the brain. With these combined approaches, we have been able to derive new mathematical algorithms (based on the mathematics of dynamical systems) that describe the neuronal mechanisms of cortical state changes and that can estimate and subtract out from the neural activity the variability in neural responses due to state changes. The algorithms for state-dependent decoding of neuronal information that we developed so far double the amount of information that we can extract from the neuronal responses, thereby demonstrating their potential for a very large improvement in the bandwidth of brain machine communication in future BMIs.
The most prominent scientific impact that we expect from SI-CODE is to push neuroscientists to rethink radically about what to measure when studying neural activity, its role in coding and brain function in general. We hope that SI-CODE will show that understanding neural computations not only requires the study of the “driver” signals reflecting neural processing of sensory or cognitive information, but also of the ongoing dynamics of the neural circuit.
SI-CODE also seeks to lay solid foundations for a long-term impact on society and technology. Our hope is that the better understanding of the state dependency of neural responses will have a direct and immediate impact on the BMIs which are currently being developed with the aim of restoring motor function to people whose brain can still deliver motor commands or intentions but the connection with actuators is non-functional. We hope that other researchers in BMI will use our open-source algorithms for state-dependent decoding of neural information immediately and improve the performance and the reliability of their devices.
In my experience, the FET Open funding scheme offers a great opportunity for scientists at all levels of seniority to pursue innovative and risky project requiring a major integration of scientific and technological disciplines on the European scale. From the personal point of view, I am particularly glad that this funding scheme allowed a pure basic scientist like me to explore the potential for his ideas for producing innovative technology. I would like to encourage especially young researchers in basic science to use this opportunity and follow their dream of contributing to major technological breakthroughs using their scientific imagination and skills.