-
3 comments
Applying the standards set by Europe’s AI High-Level Expert Group to the development of an AI-powered underwriting product
Last year, I was honoured to be chosen as the sole non-European expert to provide input, both as a Canadian and an AI tech entrepreneur, into the European Commission’s High-Level Expert Group on Artificial Intelligence (AI HLEG). In April, we published our latest version of the Ethics Guidelines for Trustworthy Artificial Intelligence.
The real success of these Guidelines has been connecting the dots from human rights to industry standards. These guidelines are grounded in a human-centric approach, informed by human and fundamental rights such as dignity, freedom, equality and justice. While there will be certain contention among some cultures and countries with some of the recommendations, I believe they are the best global reference we have for an international framework on guiding our development of AI, so far. AI isn’t contained by national borders, and ethical principles and requirements need to be rooted in concepts that promote the inherent value of all human beings, no matter their geographical location.
My role on the AI HLEG was to Co-Chair the group creating the Guidelines’ seven key requirements for AI practitioners. Each requirement does not align directly with a specific step in the development and deployment of an AI product. Rather, they serve as a lens through which to observe the whole process to assess for weaknesses and possible needs for safeguards to protect against the infringement of human rights. Below I outline how Element AI’s human-centric approach aligns with the Seven Requirements with respect to workers in insurance underwriting, and provides a means for also mitigating the impacts on consumers as well. It should be noted that there is still much work left to determine how a human-centric design will actually be used with respect to upholding the rights of end-customers in the insurance industry.
As an entrepreneur, my work in shaping the requirements would be meaningless if I didn’t also show how they manifest in our products. At my company, Element AI, we have not implemented the Requirements outright, as they’ve only just been published, but our approach echoes the same human-centric spirit in the Guidelines. In building our AI products for enterprise across multiple industries, we’ve put great effort into building our products for workers, augmenting tasks in a way that maintains their autonomy and control of outcomes.
/futurium/en/file/elementainewofficeswebres-61jpgelement_ai_new_offices_web_res-61.jpg
/futurium/en/file/elementainewofficeswebres-61jpgelement_ai_new_offices_web_res-61.jpg
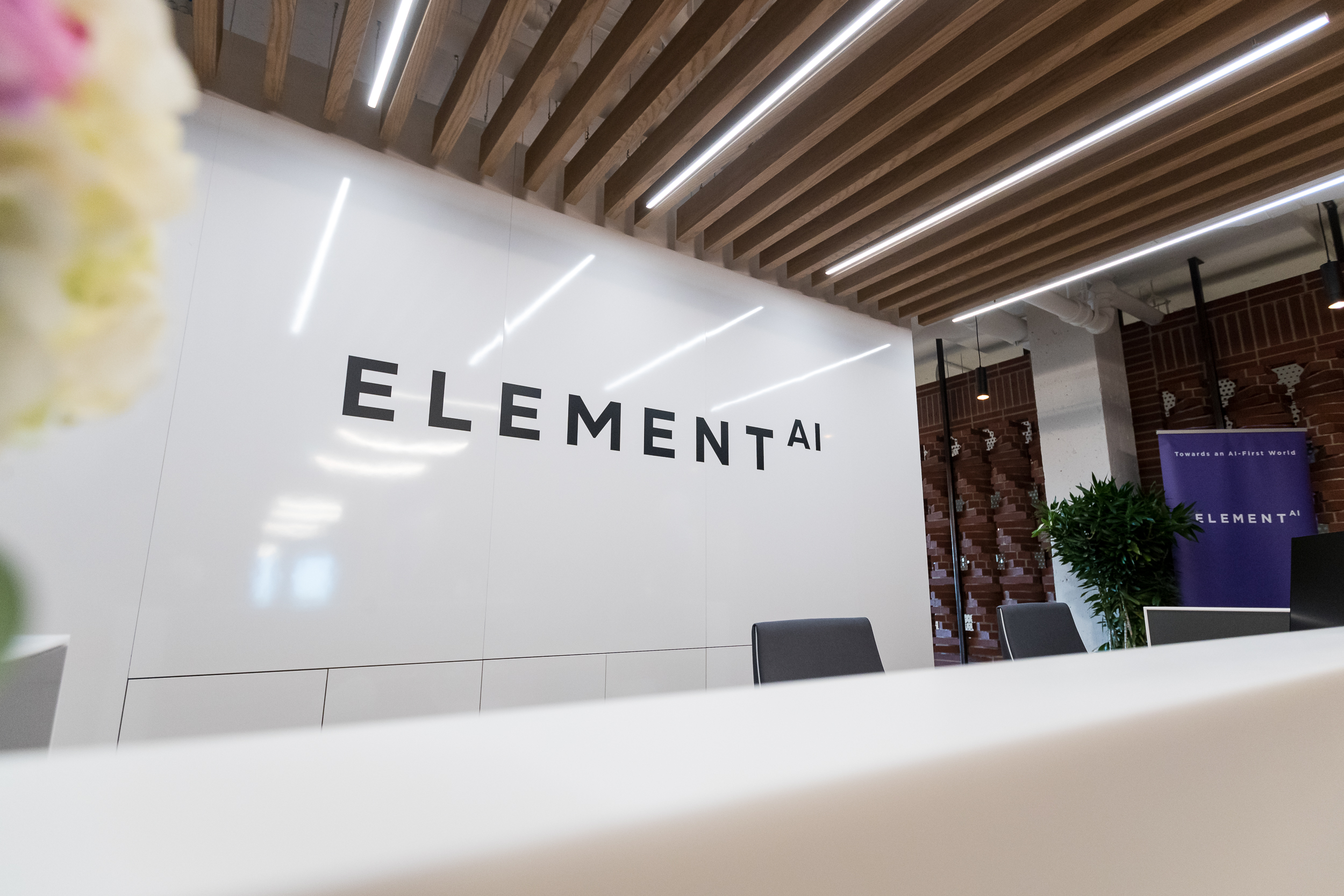
Design for the role and a human in the loop (HITL)
At Element AI, the Guidelines have reinforced the importance of human-centric AI products that support people in completing their tasks and making decisions more efficiently and with more complete information. The Guidelines’ requirements for human agency and oversight (Requirement 1), transparency (Requirement 4) and societal well-being (Requirement 6) are particularly resonant here and align with our company values and objectives. They are a valuable point of reference for how to pick the right trade offs when shaping and prioritizing features, building the interaction points for users, how the product fits into a workflow, and even where not to build a product in the first place.
In the case of our underwriting product, these requirements are rooted in our process from the beginning: the product is designed to automate low-value-added tasks and recommend decisions only where it can be trusted to meet the industry’s ethics and regulations for transparency. (Requirement 4) In supporting the role of underwriters, the carriers are able to focus on building relationships with brokers and improving interactions with customers, by explaining decisions and listening to customer needs. (Requirement 1, Requirement 6)
The tasks augmented by the product include digitizing submitted applications and, when confident enough, automatically segmenting and assigning cases to the correct underwriter. This automated decision is based on parameters set by a human administrator, including estimated processing time, urgency of case, workload, signing authority, closing ratio and more. (Requirement 1) The audit trail is also available, and provides explanations of the reasons for the segmentation and assignment of each application processed. (Requirement 4)
When making a recommendation, the AI suggests information sources that were useful for similar applications in the past, or suggests a ranked order in which to review data sources. These recommendations help underwriters prioritize information from submissions and outside sources such as databases. (Requirement 4)
When the system is not confident enough to automatically process a case, it flags it for review. It provides an explanation as to why it is not confident enough, for example closing ratio, expected processing time, or need for additional information. (Requirement 4)
The underwriter has total agency over how many applications they actually want to automatically process, for instance choosing to not automate denials-to-quote so that all denials are handled on a person-to-person level (thus preserving the underwriter/broker relationship). Even at a high level of automation, the system can flag for low-confidence predictions, high-risk cases or, if the input data is incomplete or unclear, for the underwriter to follow up on for further examination. As well, all recommendations for human-driven decisions come with levels of confidence. (Requirement 2) On the client-side, none of this changes their right to appeal decisions, whether human or automated. (Requirement 4)
The direct business value created by the product has its own societal benefit (Requirement 6): Lowering turnaround time, carriers are able to provide the right insurance to companies in the timeframe that they need, and by recommending correct coverages, our underwriting product reduces the amount of underinsured clients. (Requirement 6)
Ethics in the development of AI products
Robustness and safety checks are critical not just in how the product is designed to be used with a human in the loop, but also embedded within the build process. (Requirement 2) The confluence of data streams used in making decisions helps bring consistency in decision making by providing a more complete picture, favouring fairness and reducing bias. (Requirement 5)
Our insurance products are designed to interact with each client’s data system, and maintains the standards of privacy and data governance imposed on them by existing regulations. (Requirement 3) The product works to retain and transfer knowledge from one underwriter to another, making knowledge more accessible to junior underwriters or adjusters. (Requirement 6)
We aim to create teams of data scientists with diverse backgrounds and cultures to broaden as much as possible their ability to avoid data set biases. This helps out our insurance products provide a more fair and consistent decision to prospects. (Requirement 5) Our teams also include social scientists with backgrounds like ethics, policy and anthropology who bring an additional trained eye for spotting and addressing harmful biases and social impact. (Requirement 5, Requirement 6)
However, more solutions are needed here to be able to maintain standards across the many new scenarios of data sharing and use. We’ve collaborated with NESTA to identify suitable solutions and have focused on data trusts as a way to reinforce data governance. Data trusts could be used to give individuals more control over their personal data, as well as define the evolving concept of digital rights from a bottom-up approach. (Requirement 3, Requirement 6, Requirement 7)
The Guidelines’ 7th and final requirement is accountability, including auditability, minimisation and reporting of negative impact, trade-offs and redress. At Element AI, defining accountability with our clients is a critical process. For us, AI is not a tool that only expert builders manage; we want end users to engage with and take part in building AI, and we believe it is in accordance with the human-centricity of the Guidelines. It is challenging because practically no organization is immediately prepared to take on the new responsibilities around accountability traditionally held by the product builder. Yet with AI, it is necessary.
We provide tools trained on data sets, though they will continue to learn on new, annotated client data. The way that data is annotated will lead to different decisions and recommendations by the product, making the performance of the model dynamic (for better or for worse). With our customers, we have taken on much of the education and have developed shared agreements that clearly define whose accountability is where.
How that accountability is handled is still in an embryonic state, for both our clients and even in our own approaches as described above. It’s a function of our values and our young age as a company that we are able to apply the Guidelines in this brand new context without many legacy problems holding us back.
Next Steps
By no means do these guidelines solve it all; it’s clear that there is still much work to be done to define how the Guidelines will be applied and adopted out in the real world. That is true at Element AI as well. Mechanizing fail-safes are critical, and will likely be the toughest part to get right. The Guidelines, however, remain an extraordinary first step in having a common language and first principles to improve on as we keep innovating in our field.
Appendix: The 7 Requirements of AI Practitioners as laid out in the Ethics Guidelines for Trustworthy Artificial Intelligence.
- Human Agency and Oversight forms the first requirement, grounded in an adherence to fundamental and human rights and the necessity for AI to enable human agency.
- Technical Robustness and Safety concerns itself with the development of the AI system and focuses both on the resilience of the system against outside attacks (e.g. adversarial attacks) and failures from within, such as a miscommunication of the system’s reliability.
- Privacy and Data Governance bridges responsibilities between system developers and deployers. It addresses salient issues such as the quality and integrity of the data used in developing the AI system, and the need to guarantee privacy throughout the entire life cycle of the system.
- Transparency demands that both technical and human decisions can be understood and traced.
- Diversity, Non-Discrimination and Fairness are requirements that ensure that the AI system is accessible to everyone. These include, for example, bias avoidance, the consideration of universal design principles and the avoidance of a one-size-fits-all approach
- Societal and Environmental Well-Being is the broadest requirement and includes the largest stakeholder: our global society and the environment. It tackles the need for AI that is sustainable and environmentally friendly, as much as its impact on the democratic process.
- Accountability complements all the previous requirements, as it is relevant before, during and after the development and deployment of the AI system.